Untangling Facebook, decoding Congress: New mathematical method may help tame big data
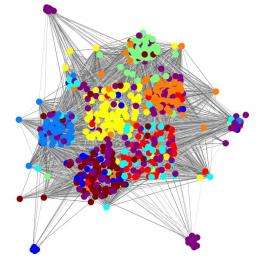
(麻豆淫院Org.com) -- Networks permeate modern life, from Facebook to political allegiances. Now University of North Carolina at Chapel Hill mathematicians and colleagues have developed a new technique for examining networks to help identify patterns and see how connections evolve. A paper describing their research appears in the May 14, 2010 edition of Science.
One of the most prominent areas of network science is the study of what鈥檚 called the 鈥渃ommunity structure鈥 of a network. But until now, key methods could only detect 鈥渃ommunities鈥 (well-connected groups of nodes) in networks that don鈥檛 change over time and only have one type of connection.
Of course, most networks in real life are more complicated, said Peter J. Mucha, Ph.D., associate professor of mathematics in the UNC College of Arts and Sciences and lead author of the paper. The new technique offers the ability to examine networks that vary over time and have multiple kinds of connections.
鈥淚t鈥檚 鈥榗onnecting the dots鈥 on steroids,鈥 Mucha said. 鈥淭his method offers new potential for handling a fire hose of information, whether you鈥檙e looking at an online social network or a real-world web of people or things.鈥
Mucha and his colleagues derived their new method from mathematical principles and applied it to a few example datasets, including the complete historical roll call voting record in the U.S. Senate through 2008, and a set of Facebook profiles from almost 1,700 students at an anonymous American university including photo tags and housing information. Mucha said their community detection methodology identified some interesting details, including points of historical transition in the Senate and indications of different groups among Facebook users.
鈥淔acebook is a good example of a tangled web of connections,鈥 he said. 鈥淲ithin it, there are groups of people who are more tightly connected to each other than they are to other groups. If you map out every individual 鈥榝riend鈥 connection and trace one connection to another, you鈥檒l see some clumpiness to that network.鈥
But a more complete analysis of the network would include information about the myriad of different types of connections. For example, by analyzing data such as individuals鈥 profile details, photo tags, Facebook 鈥渓ikes鈥 and recommendations and messages, it might be possible to identify other connections and groups that may be subtle or not explicitly obvious, Mucha said. (The paper in Science did not look at all such information)
The new method divides a network into multiple 鈥渟lices,鈥 with each slice representing the network at one snapshot in time, or a different set of connections between the individuals within it. These slices are then combined and - by using a variety of computer algorithms - analyzed to identify communities.
Mucha鈥檚 primary interest in network analysis is applying methodologies to real world data, including congressional relationships.
With the new community detection method, researchers should be able to dig deeper to examine the relationships among different groups in dynamic, multiplex data. Identifying community structures in a network might help to model processes and provides a signal about the underlying system, such as legislative polarization or the influence of various factors and forces, he said.
鈥淟ooking at the way legislators vote, it鈥檚 usually easy to quickly group them into Republicans and Democrats, but that鈥檚 really just a first pass at the data,鈥 he said. 鈥淭hose legislators might be connected in many ways 鈥 the states they represent, who they鈥檝e received political donations from, their caucuses or committee assignments, even where their offices are located in the building. Combining such information in a meaningful way helps us explore - and potentially make more sense of - legislative data.鈥
Mucha believes another potential application for the new method is modeling the spread of diseases. He plans new research in that area.
Two UNC undergraduates - Thomas Richardson, class of 2008, and Kevin Macon, class of 2010 - are among the paper鈥檚 co-authors, along with Mason A. Porter of Oxford University and Jukka-Pekka Onnela of Harvard University.
Provided by University of North Carolina at Chapel Hill