Researchers find six model parameters individually influence precipitation in a global model
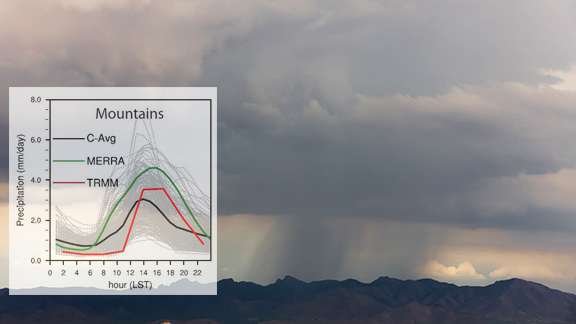
When crunched, climate model numbers can fluctuate depending on which climate components are examined. To quantify this uncertainty, researchers at Pacific Northwest National Laboratory systematically identified six modeling parameters that have the greatest influence on modeling global precipitation. They found that the influence of individual parameters does not depend on sampling approach or the associated parameters.
By using Uncertainty Quantification (UQ) tools, they showed that the precipitation variance is caused by contributions from individual parameters most of the time. Further, the variation is seasonally influenced significantly in mid-latitude regions—not so much in tropical continental regions.
The Earth's climate involves complex interactions among ever-changing physical components and chemical processes. There are myriad ways that clouds form and uncounted conditions that provoke precipitation. Climate researchers work to break down those processes and components into simpler representations so that computers can be used to understand how those processes interact. Usually, climate modelers "tune" these representations, called parameters, one at a time and then assume the response in the model is related to that individual parameter.
The researchers in this study found that precipitation does not always respond to individual parameter tuning in this way. Further, they found that certain parameters influence independently, rather than through interactions among them, to produce much of the precipitation variance in the models. These findings lead the researchers to investigate parameter tuning that considers each component's physics, without having to consider compensating errors from other parameters. Their work increases confidence in more targeted studies that focus on a single parameter and its effects on precipitation.
A research team led by PNNL used two model sampling approaches (the Latin hypercube sampling and quasi-Monte Carlo) to explore the high-dimensional (wide variance) parameter space and identify the most influential parameters. They conducted two large sets of simulations: 1100 simulations in the cloud ensemble focused on 22 parameters related to cloud and convection processes, and 256 simulations in the aerosol ensemble focused on 16 parameters related to aerosol and cloud microphysics. They also applied a surrogate model (generalized linear model) to provide quantitative measures of how sensitive the model output is to uncertain parameters.
"While this study only focused on the response and sensitivity of precipitation to model parameters, we demonstrated an approach that can be easily applied to other variables of interest," said Dr. Yun Qian, PNNL atmospheric modeler and lead author of the study.
This approach will guide the next step to reducing a model's precipitation uncertainty by calibrating the most uncertain parameters and developing new model parameterizations for those components.
The parameter tuning process in global climate models is often optimized to minimize the difference between model fields and observations, such as precipitation. This process may produce a set of parameter settings that approximate the observed climate but this may not affect physical processes that lead to the climate. In future studies, the work suggests quantifying the sensitivity of parameters describing physical processes (i.e., turbulence, shallow and deep convection process) for model calibration.
More information: "Parametric Sensitivity Analysis of Precipitation at Global and Local Scales in the Community Atmosphere Model CAM5." Journal of Advanced Modeling Earth Systems.
Provided by Pacific Northwest National Laboratory