September 23, 2019 feature
Unfolding adsorption on metal nanoparticles: Connecting stability with catalysis
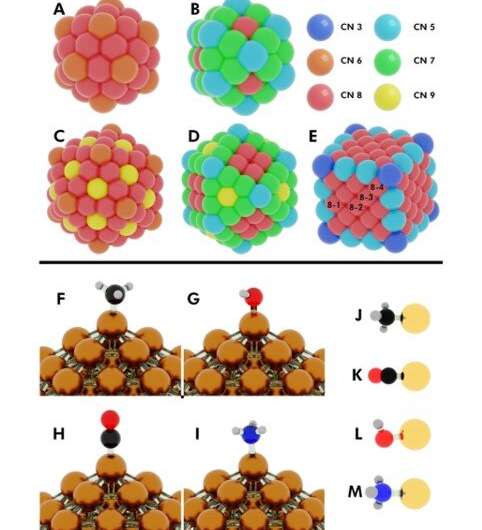
Metal nanoparticles have received substantial attention due to their applications in diverse fields from medicine, catalysis, energy and the environment. However, the fundamental properties of nanoparticle adsorption on a surface remain to be understood. James Dean and an interdisciplinary research team in the department of Chemical Engineering, in the U.S. introduced a universal adsorption model to account for the structural characteristics, metal composition and different adsorbates of nanoparticles via machine learning (ML). The model fit a large number of data to accurately predict adsorption trends on monometallic and alloy-based nanoparticles. The template was simple and provided rapidly calculated data for metals and adsorbates. The research team connected the adsorption with stability behavior to advance the design of optimal nanoparticles for applications of interest. The research is now published on Science Advances.
Metal nanoparticles (NPs) have significant applications in catalysis, ranging from , to . But their stability and catalytic activity generally show opposing trends, where very active catalysts can only operate for a few cycles. A key feature on the extent of metallic catalytic functionality depends on the strength of adsorption for a variety of species on the catalyst surface. According to the , developed more than a century ago, active catalysts should bind adsorbates with a binding strength that is neither strong nor weak. While strongly adsorbed species can poison the catalyst surface, weakly bound reactants desorb easily. In an intermediate scenario, the reactants can meet each other and react on the catalytic surfaces. Researchers currently use and theoretical chemistry methods to stimulate catalytic behavior on metal catalysts with great accuracy to guide subsequent experiments in the lab.
Computational efforts have focused on screening different metal catalysts to discover the "magic" binding energy (BE) of chemical species on catalyst surfaces to form very active catalysts. The in silico design of catalytically active materials, however, remains to be realized. The drawbacks are mainly due to design efforts that often neglect the stability of catalysts. NP catalysts also possess a high degree of site heterogeneity on their surface for adsorption and catalysis. Scientists had developed adsorption models to relate binding energy of the adsorbates with surface characteristics of NPs such as (CNs) to understand the site-specific adsorption response. Yet, for clarity, the binding energy (BE) variation also entails secondary descriptors such as and of NPs.
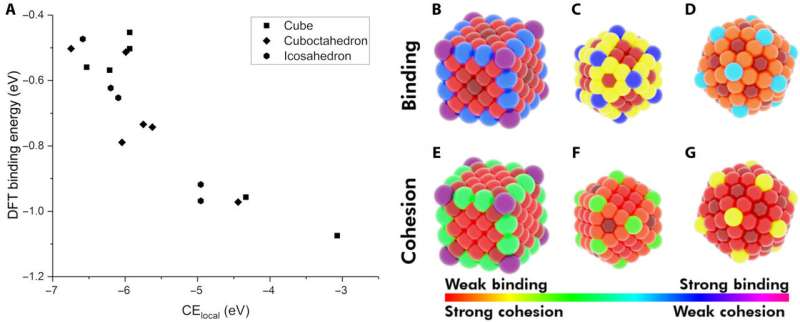
In the present work, Dean et al. applied (DFT) and machine learning techniques to derive a simple physics-based model to accurately capture the varying adsorption energy. They estimated the variable as a function of the local adsorption site environment on the NP surface as well as the type of metal NP. The generalized model could be applied to any metal nanostructure to understand adsorption behavior on the NP catalyst and the stability of the catalyst; to screen and .
The researchers first hypothesized the most important factors between monometallic NPs and adsorbates. They then defined the (CElocal) in bulk metals and captured CEs in NPs using a , which summed every metal-metal bond energy. By applying similar concepts, they described the stability of binding sites and showed how chemically unsaturated sites (fewer metal-metal bonds) bound adsorbates with an increased strength. The research team focused on describing the binding capacity of a single adsorbate-metal pair. They plotted the DFT-calculated binding energy of carbon monoxide (CO) to a 172-atom gold (Au) cube and a 147-atom gold (Au) cuboctahedron or icosahedron. The team observed a strongly inverse relationship between the local cohesive energy (CElocal) and binding energy (BE) to suggest the strongest adsorption sites to be those exhibiting the weakest local cohesion.
The team further developed their model and performed (OLS) regression to understand adsorption on monometallic NPs and slabs using three adsorbates [Methyl radical (CH3), CO, hydroxyl radical (OH)] on three different metals (Cu, Ag—silver, Au). The metallic NPs contained different morphologies (172-atom cube, 55- and 147-atom icosahedron and 55- and 147-atom cuboctahedron). They observed that the binding affinity to the adsorbates decreased as the cohesion of the local sites increased. And as the adsorbate's chemical potential increased, they became less stable and bound a metal NP with higher tendency. The direct correlation with the metal Adsorbate (MAD) intuitively described the tendency of the metal to bind the adsorbate.
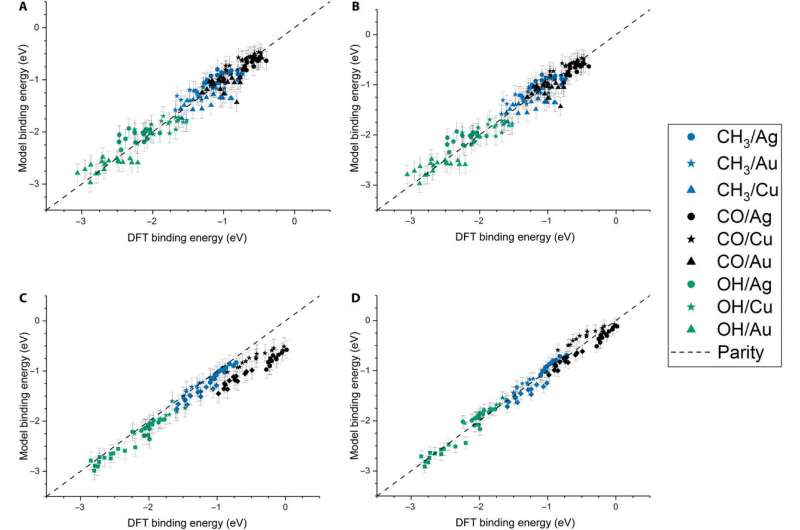
Dean et al. tested the generalizability of the model and trained the simulation on a single metal or single morphology, although it accurately captured other metals or morphologies as well. The work provided strong evidence that the model captured the underlying physics of the binding interactions, allowing the team to extend the work from non-periodic NPs to periodic slab systems. Computationally inexpensive systems could parameterize the model to extend to larger systems, which was not thus far possible due to the computational costs involved.
Dean et al. then extended the model from monometallic NPs to bimetallic systems. For these experiments, they plotted the BE—trained on monometallic NPs, across several sites of bi-metallic, 55-atom icosahedron NPs (Cu31Ag24 and Cu22Ag33). The model very accurately captured trends in adsorption on the bimetallic Cu/Ag NPs as well. This was an interesting result since the scientists had only trained the model on monometallic systems. The results showed the generalizability of the model for both monometallic and bimetallic NPs. However, the team will account additional descriptors including binding site electronegativity to understand the adsorption behavior for bimetallic systems in depth.
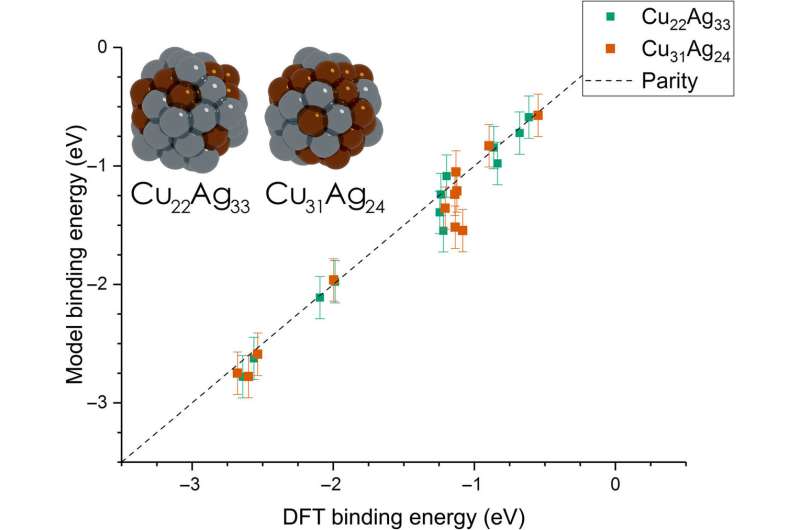
Although Dean et al. trained the ML (machine learning) algorithm to capture the adsorption trends of just one type of , it could accurately predict the behavior of similar d9 metals (Cu—coper, Ag and Au). When they trained the model on a dataset of CH3, CO and OH adsorbed to Cu, Ag and Au NPs, they could also capture general adsorption trends for similar elements in other columns of the periodic table. They then improved the complexity of the machine learning techniques to provide additional avenues to improve the model of adsorption.
In this way, James Dean and his colleagues developed a simple yet powerful physics-based model to capture trends on the strength of binding interactions between different adsorbates and metal NPs using machine learning techniques. The study was the first to develop an adsorption model that accurately connected the properties of diverse metal NPs with the stability of the adsorption site. The model introduced simple descriptors to capture the adsorption on any site, relative to monometallic and bimetallic NPs. The team generalized the model to effectively stimulate a range of binding interactions, including variations on the types of metals, their composition, sites of adsorption and adsorbates.
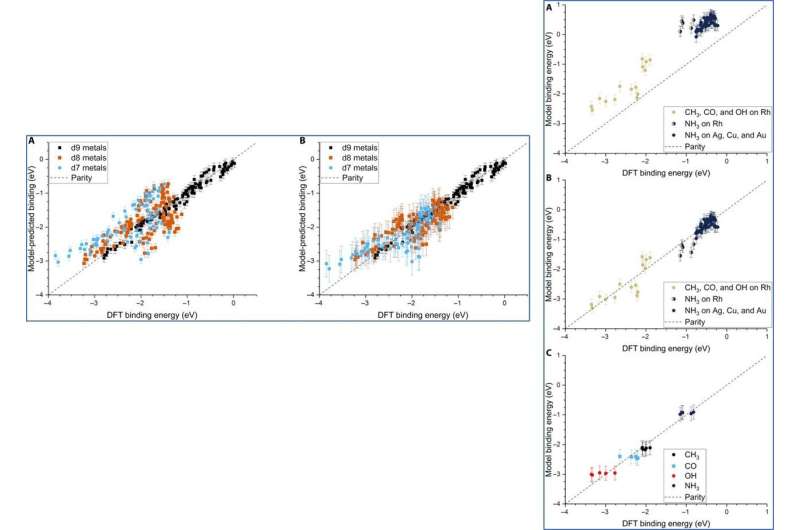
Although the team did not test the applicability of the model for ternary systems, the physical properties may remain relevant to accurately model multimetallic systems as well. The adsorption model can accurately describe the binding strength of a variety of molecules on any site of NPs, including alloys. The scientists expect the model to be highly applicable as a screening tool for the high throughput search of potential catalysts.
More information: James Dean et al. Unfolding adsorption on metal nanoparticles: Connecting stability with catalysis, Science Advances (2019).
M. Valden. Onset of Catalytic Activity of Gold Clusters on Titania with the Appearance of Nonmetallic Properties, Science (2002).
Jeff Greeley et al. Alloy catalysts designed from first principles, Nature Materials (2004).
Journal information: Science Advances , Nature Materials , Science
© 2019 Science X Network