A new model for measuring global water storage
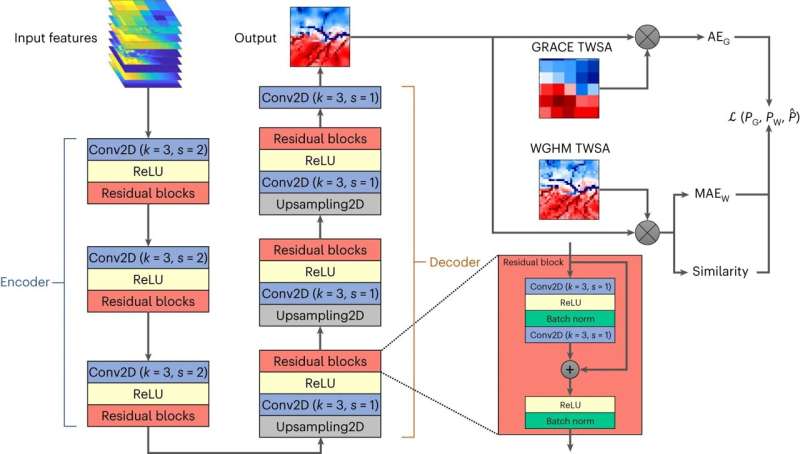
In their recent in Nature Water, D-BAUG researchers Junyang Gou and Professor Benedikt Soja introduced a finely resolved model of terrestrial water storage using a novel deep learning approach.
By integrating satellite observations with hydrological models, their method achieves remarkable accuracy even in smaller basins.
This model promises significant benefits across various domains, including hydrology, climate science, sustainable water management, and hazard prediction.
More information: Junyang Gou et al, Global high-resolution total water storage anomalies from self-supervised data assimilation using deep learning algorithms, Nature Water (2024).
Coomentary: Alexander Sun, Learning to downscale satellite gravimetry data through artificial intelligence, Nature Water (2024).
Journal information: Nature Water
Provided by ETH Zurich